Ai & Machine Ops
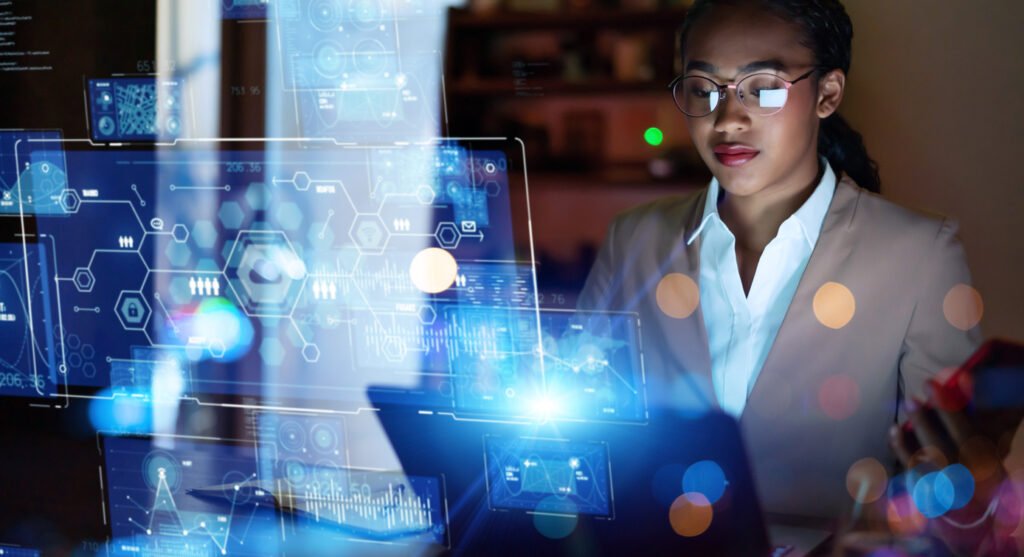
AI and Machine Learning Operations (MLOps): The New Frontier in Business
In the ever-evolving landscape of business technology, AI and Machine Learning Operations (MLOps) stand at the forefront of transforming business operations. MLOps, the integration of machine learning and operations, focuses on the deployment, monitoring, and maintenance of AI systems in real-world business settings. This approach is essential for the effective functioning of AI models, ensuring they deliver optimal performance and value in various industry applications. As businesses increasingly rely on AI-driven processes, the role of MLOps becomes pivotal in bridging the gap between AI development and operational execution.
Importance of MLOps in Modern Business
MLOps is vital for ensuring that AI systems operate efficiently and effectively. It involves a combination of data science, DevOps, and machine learning, and is focused on the end-to-end lifecycle of AI and machine learning in the business environment. By adopting MLOps, businesses can streamline the deployment and management of AI models, enabling faster time-to-market and continuous improvement of their AI solutions.
Real-World Scenarios and Applications
- Retail Industry: In retail, MLOps can optimize inventory management, predicting stock requirements based on consumer behavior analysis. By continuously monitoring and updating the AI models, retailers can adapt to changing trends and demands, reducing waste and increasing sales.
- Healthcare Sector: MLOps plays a crucial role in healthcare by maintaining AI systems used for patient diagnosis and treatment plans. Continuous monitoring ensures the accuracy and reliability of these AI systems, which is critical in life-saving scenarios.
- Finance and Banking: In finance, MLOps is used for fraud detection systems. It helps in the constant updating and tuning of AI models to identify new fraudulent patterns, protecting both the institution and its customers.
Challenges in Implementing MLOps
Skills Gap
The implementation of MLOps requires a unique blend of skills:
- Data Science Expertise: Understanding of machine learning algorithms and data analysis.
- DevOps Knowledge: Skills in software development and operations, focusing on continuous integration and delivery.
- System Architecture Understanding: Knowledge of how to design and manage scalable and efficient systems.
Security in MLOps
Security concerns in MLOps revolve around:
- Data Protection: Ensuring the confidentiality and integrity of data used in AI models.
- Model Security: Safeguarding AI models against tampering and unauthorized access.
- Compliance: Adhering to data protection regulations like GDPR.
Addressing these challenges involves comprehensive training programs, hiring specialists, and implementing robust security protocols and compliance measures. These steps are essential for the secure and effective application of MLOps in business environments.
Future of MLOps in Business
The future of MLOps is promising, with advancements in AI technologies leading to more sophisticated and efficient operational models. Businesses that embrace MLOps are likely to stay ahead in the competitive market by leveraging the full potential of AI and machine learning. As the adoption of AI continues to grow, MLOps will become an indispensable component of successful AI implementations, ensuring that businesses can reap the benefits of this transformative technology. MLOps represents a new frontier in business technology, offering a structured approach to managing AI systems in various industries. By adopting MLOps, businesses can maximize the efficiency and effectiveness of their AI investments, driving innovation and gaining a competitive edge in the market.
References
https://cloud.google.com/mlops
https://www.ibm.com/topics/mlops
https://ml-ops.org/
https://www.retaildive.com/news/how-ai-and-machine-learning-are-transforming-retail/559839/
https://www.nature.com/articles/s41746-022-00625-9
https://www.forbes.com/sites/forbestechcouncil/2022/03/31/how-mlops-is-transforming-the-financial-services-industry/
https://www.oreilly.com/radar/the-mlops-skills-gap/
https://www.microsoft.com/en-us/security/business/mlops-security
https://www.mckinsey.com/capabilities/mckinsey-digital/our-insights/mlops-industrializing-ai
AI and Machine Learning Operations (MLOps): The New Frontier in Business
In the ever-evolving landscape of business technology, AI and Machine Learning Operations (MLOps) stand at the forefront of transforming business operations. MLOps, the integration of machine learning and operations, focuses on the deployment, monitoring, and maintenance of AI systems in real-world business settings. This approach is essential for the effective functioning of AI models, ensuring they deliver optimal performance and value in various industry applications. As businesses increasingly rely on AI-driven processes, the role of MLOps becomes pivotal in bridging the gap between AI development and operational execution.
Importance of MLOps in Modern Business
MLOps is vital for ensuring that AI systems operate efficiently and effectively. It involves a combination of data science, DevOps, and machine learning, and is focused on the end-to-end lifecycle of AI and machine learning in the business environment. By adopting MLOps, businesses can streamline the deployment and management of AI models, enabling faster time-to-market and continuous improvement of their AI solutions.
Real-World Scenarios and Applications
- Retail Industry: In retail, MLOps can optimize inventory management, predicting stock requirements based on consumer behavior analysis. By continuously monitoring and updating the AI models, retailers can adapt to changing trends and demands, reducing waste and increasing sales.
- Healthcare Sector: MLOps plays a crucial role in healthcare by maintaining AI systems used for patient diagnosis and treatment plans. Continuous monitoring ensures the accuracy and reliability of these AI systems, which is critical in life-saving scenarios.
- Finance and Banking: In finance, MLOps is used for fraud detection systems. It helps in the constant updating and tuning of AI models to identify new fraudulent patterns, protecting both the institution and its customers.
Challenges in Implementing MLOps
Skills Gap
The implementation of MLOps requires a unique blend of skills:
- Data Science Expertise: Understanding of machine learning algorithms and data analysis.
- DevOps Knowledge: Skills in software development and operations, focusing on continuous integration and delivery.
- System Architecture Understanding: Knowledge of how to design and manage scalable and efficient systems.
Security in MLOps
Security concerns in MLOps revolve around:
- Data Protection: Ensuring the confidentiality and integrity of data used in AI models.
- Model Security: Safeguarding AI models against tampering and unauthorized access.
- Compliance: Adhering to data protection regulations like GDPR.
Addressing these challenges involves comprehensive training programs, hiring specialists, and implementing robust security protocols and compliance measures. These steps are essential for the secure and effective application of MLOps in business environments.
Future of MLOps in Business
The future of MLOps is promising, with advancements in AI technologies leading to more sophisticated and efficient operational models. Businesses that embrace MLOps are likely to stay ahead in the competitive market by leveraging the full potential of AI and machine learning. As the adoption of AI continues to grow, MLOps will become an indispensable component of successful AI implementations, ensuring that businesses can reap the benefits of this transformative technology.MLOps represents a new frontier in business technology, offering a structured approach to managing AI systems in various industries. By adopting MLOps, businesses can maximize the efficiency and effectiveness of their AI investments, driving innovation and gaining a competitive edge in the market.
References
:https://cloud.google.com/mlops
https://www.ibm.com/topics/mlops
https://ml-ops.org/
https://www.retaildive.com/news/how-ai-and-machine-learning-are-transforming-retail/559839/
https://www.nature.com/articles/s41746-022-00625-9
https://www.forbes.com/sites/forbestechcouncil/2022/03/31/how-mlops-is-transforming-the-financial-services-industry/
https://www.oreilly.com/radar/the-mlops-skills-gap/
https://www.microsoft.com/en-us/security/business/mlops-security
https://www.mckinsey.com/capabilities/mckinsey-digital/our-insights/mlops-industrializing-ai